Unlocking Python's Levenshtein Library: A Guide to String Similarity
Written on
Chapter 1: Introduction to the Python Levenshtein Library
The Python Levenshtein library is a powerful tool designed for calculating the Levenshtein distance between two strings. This distance, often referred to as the edit distance, quantifies the minimum number of edits—insertions, deletions, or substitutions—required to convert one string into another. The library offers a highly efficient implementation of this algorithm, making it useful across a variety of applications.
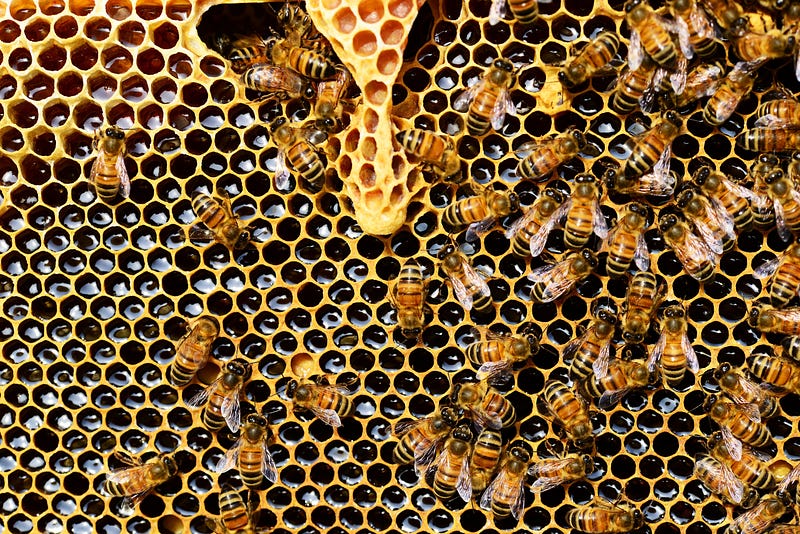
Chapter 1.1: Applications of Levenshtein Distance
One prevalent application of the Levenshtein distance is in spell checking and natural language processing. By measuring the distance between a misspelled term and a database of correctly spelled words, the library can identify the closest match and recommend a correction. This methodology is also applicable in other fields, such as genetics, where it helps compare DNA sequences to find similarities and discrepancies.
Additionally, the Levenshtein distance plays a crucial role in information retrieval and search engines. By evaluating how closely a query matches a collection of documents, it can rank search results based on their relevance, enhancing the accuracy of returned information.
Section 1.2: Using the Python Levenshtein Library
Integrating the Levenshtein library into your Python projects is a simple process. To begin, install the library by executing the command “pip install python-Levenshtein” in your terminal. Once the installation is complete, you can import the library into your Python script.
Here’s a quick example demonstrating how to calculate the Levenshtein distance between two strings:
import Levenshtein
string1 = "kitten"
string2 = "sitting"
distance = Levenshtein.distance(string1, string2)
print(distance)
This code snippet will yield an output of 3, indicating that three operations are necessary to convert “kitten” to “sitting” (changing k to s, e to i, and n to g).
Chapter 2: Additional Features of the Library
Beyond the distance function, the Python Levenshtein library includes several other useful functions, such as ratio() and hamming(), which cater to various scenarios and needs.
The first video titled "NLP 02: String Similarity, Cosine Similarity, Levenshtein Distance" delves into the concepts of string similarity, providing valuable insights into these algorithms and their applications.
The second video, "Mastering Address Matching in Excel with FuzzyMatch Logic," offers practical strategies for implementing fuzzy matching techniques in Excel, showcasing the utility of string similarity concepts in real-world scenarios.
In summary, the Python Levenshtein library is an exceptional resource for evaluating string similarity. Its applications span spell checking, natural language processing, information retrieval, and numerous other fields. With its user-friendly API and efficient design, it is an indispensable tool for data scientists and developers alike.